Clea Parcerisas
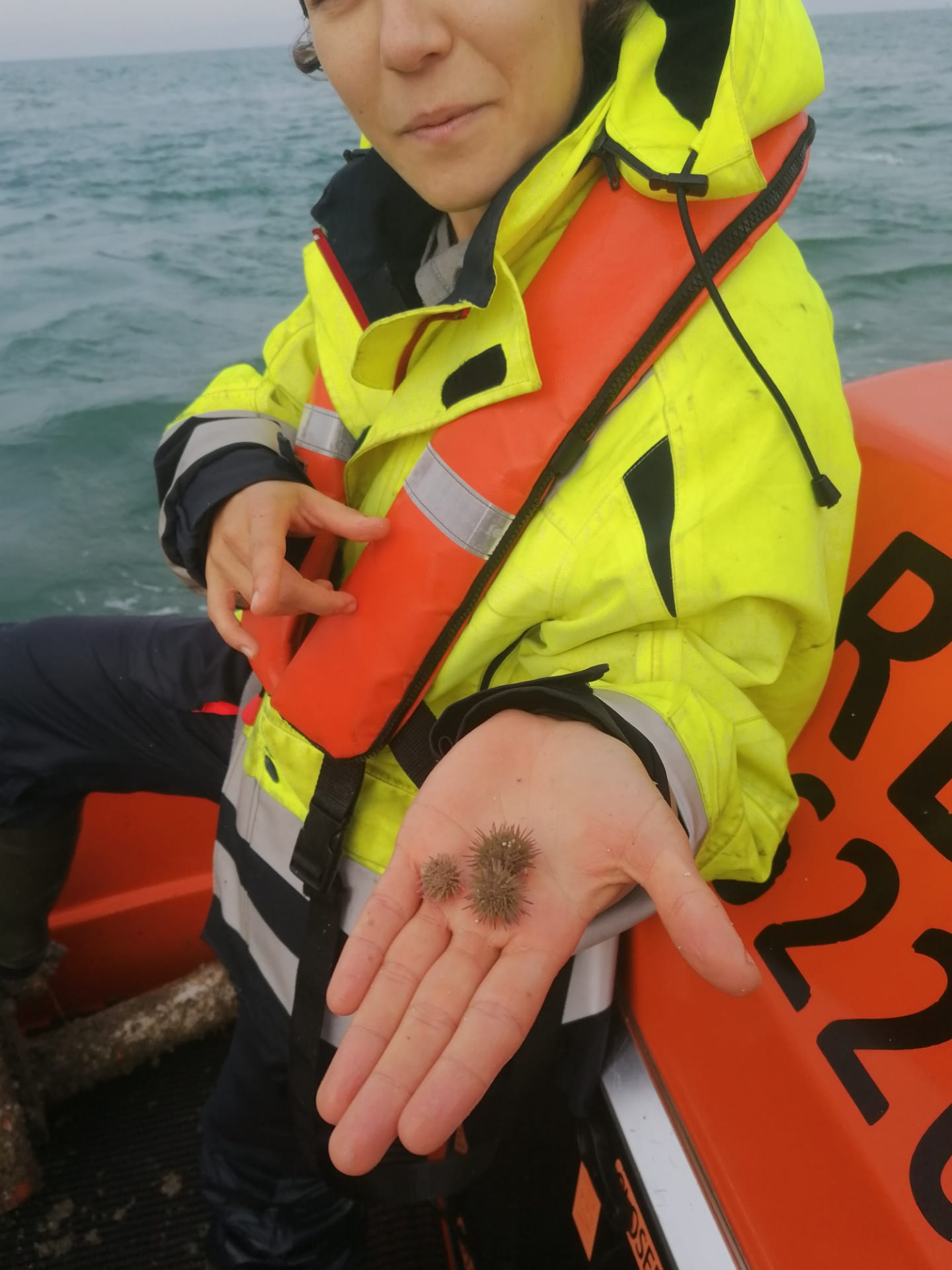
I am a post-doc researchers at the Flanders Marine Institute (VLIZ), in Belgium.
I recently received my PhD at Ghent University (and VLIZ) in June 2024, under the supervision of Prof. Dick Botteldooren, Prof. Paul Devos and Dr. Elisabeth Debusschere.
I like developing open-source tools for marine bioacoustics analysis.
selected publications
- Comparison of the effects of reef and anthropogenic soundscapes on oyster larvae settlementSarah Schmidlin, Clea Parcerisas, Jeroen Hubert, and 6 more authorsScientific Reports, May 2024
Settlement is a critical period in the life cycle of marine invertebrates with a planktonic larval stage. For reef-building invertebrates such as oysters and corals, settlement rates are predictive for long-term reef survival. Increasing evidence suggests that marine invertebrates use information from ocean soundscapes to inform settlement decisions. Sessile marine invertebrates with a planktonic stage are particularly reliant on environmental cues to direct them to ideal habitats. As gregarious settlers, oysters prefer to settle amongst members of the same species. It has been hypothesized that oyster larvae from species Crassostrea virginica and Ostrea angasi use distinct conspecific oyster reef sounds to navigate to ideal habitats. In controlled laboratory experiments we exposed Pacific Oyster Magallana gigas larvae to anthropogenic sounds from conspecific oyster reefs, vessels, combined reef-vessel sounds as well as off-reef and no speaker controls. Our findings show that sounds recorded at conspecific reefs induced higher percentages of settlement by about 1.44 and 1.64 times compared to off-reef and no speaker controls, respectively. In contrast, the settlement increase compared to the no speaker control was non-significant for vessel sounds (1.21 fold), combined reef-vessel sounds (1.30 fold), and off-reef sounds (1.18 fold). This study serves as a foundational stepping stone for exploring larval sound feature preferences within this species.
- Machine learning for efficient segregation and labeling of potential biological sounds in long-term underwater recordingsClea Parcerisas, Elena Schall, Kees Velde, and 3 more authorsFrontiers in Remote Sensing, Apr 2024
Studying marine soundscapes by detecting known sound events and quantifying their spatio-temporal patterns can provide ecologically relevant information. However, the exploration of underwater sound data to find and identify possible sound events of interest can be highly time-intensive for human analysts. To speed up this process, we propose a novel methodology that first detects all the potentially relevant acoustic events and then clusters them in an unsupervised way prior to manual revision. We demonstrate its applicability on a short deployment. To detect acoustic events, a deep learning object detection algorithm from computer vision (YOLOv8) is re-trained to detect any (short) acoustic event. This is done by converting the audio to spectrograms using sliding windows longer than the expected sound events of interest. The model detects any event present on that window and provides their time and frequency limits. With this approach, multiple events happening simultaneously can be detected. To further explore the possibilities to limit the human input needed to create the annotations to train the model, we propose an active learning approach to select the most informative audio files in an iterative manner for subsequent manual annotation. The obtained detection models are trained and tested on a dataset from the Belgian Part of the North Sea, and then further evaluated for robustness on a freshwater dataset from major European rivers. The proposed active learning approach outperforms the random selection of files, both in the marine and the freshwater datasets. Once the events are detected, they are converted to an embedded feature space using the BioLingual model, which is trained to classify different (biological) sounds. The obtained representations are then clustered in an unsupervised way, obtaining different sound classes. These classes are then manually revised. This method can be applied to unseen data as a tool to help bioacousticians identify recurrent sounds and save time when studying their spatio-temporal patterns. This reduces the time researchers need to go through long acoustic recordings and allows to conduct a more targeted analysis. It also provides a framework to monitor soundscapes regardless of whether the sound sources are known or not.
- Deep learning in marine bioacoustics: a benchmark for baleen whale detectionElena Schall, Idil Ilgaz Kaya, Elisabeth Debusschere, and 2 more authorsRemote Sensing in Ecology and Conservation, Apr 2024
Passive acoustic monitoring (PAM) is commonly used to obtain year-round continuous data on marine soundscapes harboring valuable information on species distributions or ecosystem dynamics. This continuously increasing amount of data requires highly efficient automated analysis techniques in order to exploit the full potential of the available data. Here, we propose a benchmark, which consists of a public dataset, a well-defined task and evaluation procedure to develop and test automated analysis techniques. This benchmark focuses on the special case of detecting animal vocalizations in a real-world dataset from the marine realm. We believe that such a benchmark is necessary to monitor the progress in the development of new detection algorithms in the field of marine bioacoustics. We ultimately use the proposed benchmark to test three detection approaches, namely ANIMAL-SPOT, Koogu and a simple custom sequential convolutional neural network (CNN), and report performances. We report the performance of the three detection approaches in a blocked cross-validation fashion with 11 site-year blocks for a multi-species detection scenario in a large marine passive acoustic dataset. Performance was measured with three simple metrics (i.e., true classification rate, noise misclassification rate and call misclassification rate) and one combined fitness metric, which allocates more weight to the minimization of false positives created by noise. Overall, ANIMAL-SPOT performed the best with an average fitness metric of 0.6, followed by the custom CNN with an average fitness metric of 0.57 and finally Koogu with an average fitness metric of 0.42. The presented benchmark is an important step to advance in the automatic processing of the continuously growing amount of PAM data that are collected throughout the world’s oceans. To ultimately achieve usability of developed algorithms, the focus of future work should be laid on the reduction of the false positives created by noise.